The next industrial revolution will require the emergence of brand-new engineering discipline, one that will be data-driven, computationally intensive, biology-inspired and human-centric.
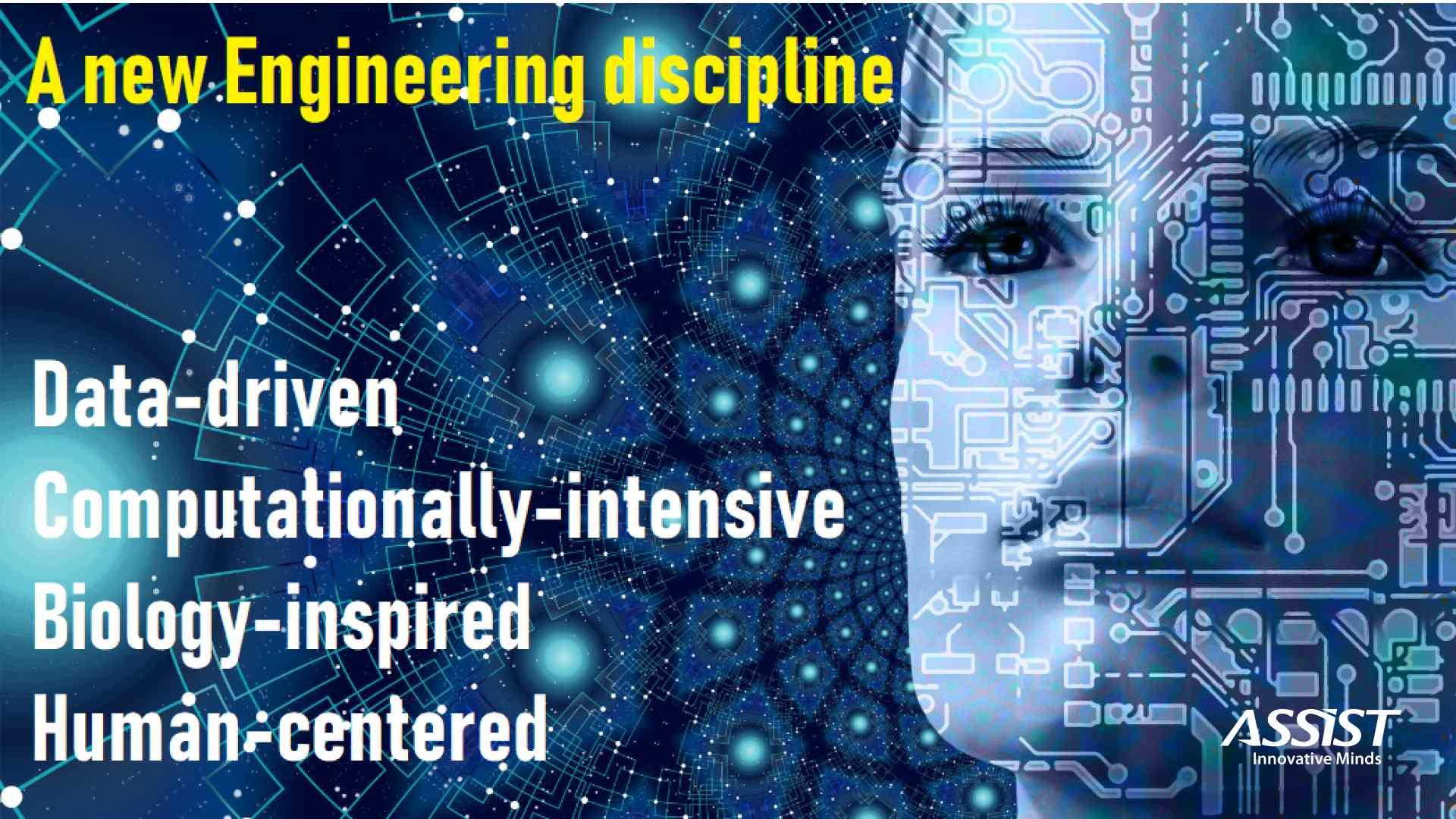
Credit to Google images for background picture
We are currently witnessing the onset of a new paradigm that is going to revolutionize in a fundamental way the Science, Engineering, and Technology of tomorrow. This paradigm implies the consolidated use of theory, experimentation, and simulation, and is driven by the availability of extremely large amounts of data generated as a result of great technological innovations.
This is the dawn of a new era where the process of decision-making is going to be driven by data and powered by algorithms. This emerging paradigm [1] is very different from the ones that proceeded because is the result of the convergence of various models and archetypes from a variety of domains. This innovative approach is going to fundamentally change the landscape in which many industries will operate in the future, and it will particularly revolutionize the fashion in which the future workforce will be structured and prepared for the challenges of the next industrial revolution.
Nature does not fit itself very well into the rigorous constraints imposed by Math, Physics or Computer Engineering. But instead, the natural world juggles between disciplinary boundaries to achieve its main objectives – survival and evolution.
As the general body of knowledge is becoming ever more complex, so does the requirement for a higher threshold of scientific competence and greater interdisciplinarity among researchers and practitioners. This article will bring to the attention of the reader the emergence of a new engineering discipline that, in my opinion, is going to have a powerful impact not only on the future of the financial industry but more generally on any other sectors of the modern economy.
1. The Problem
As someone who was engaged for more than two decades in the field of quantitative and computational finance, from both an engineering and quant perspective, I especially appreciate the importance of higher education and professional training. The most important lesson that I learned in my career, is that the potential to solve complex business problems depends predominantly on being able to recruit, train and develop the necessary talent that could apply effectively the latest technology available.
The global markets for talent recruiting and management are extremely large, estimated at about $250-300B annually. Their identified inefficiencies are so large in scope and so critical to the success of the next industrial revolution, that they require the adoption of a revolutionary new paradigm-shifting the emphasis from content acquisition to experiential learning at a very accelerated pace.
There is a widespread perception that the current higher education system is technically outdated, costly, inefficient and often irrelevant to the needs of modern industries, and the financial industry is no exception. The current models are in dire need of feedback from an industry where recruiting and continuous education has become very costly and inefficient. While business is spending massive amounts of resources on recruiting and training, the learners are incurring ever-increasing costs to attend educational programs that are not meeting today’s standards.
Educating the 21st-century hi-tech workforce will require a brand new approach, one that is more relevant, modern and efficient, for both the employers and their current and future workforce.
Current research in Neuro-Psychology, human Cognition and Cognitive Computing, points to the fact that the learning process should not be dedicated principally to content acquisition, but it should be rather focused on how to efficiently apply the acquired knowledge to solve practical problems.
The efficiency of the learning process should be quantifiable, in ways that will allow actionability to be measured and optimized. Today’s generation of quants and financial engineers is facing brand new challenges as new skillsets and know-how are required from them. The life-cycle of modern technologies is averaging now about 2.5 years, but it will become certainly shorter in the near future. Everyone should be ready to embrace and thrive in a life-long cycle of learning.
This is a new era, one of risk aversion and frugality where new expectations are emerging for the workforce. This dynamic has created the conditions for the emergence of a new engineering discipline, one that will be centered on human abilities and needs, deeply rooted in Biology concepts, while being Data-driven and Computationally-intensive.
By integrating a variety of quantitative, computational and life science approaches, this new engineering discipline is called to break the barriers that keep us from reaching the AI-dream, having as principal objective to enhance human life.
2. Solution Drivers
2.1 The Great Convergence
The last two decades have seen the parallel evolution of two distinct, yet related branches in several major scientific disciplines. As one of the oldest scientific disciplines, Medicine is currently developing novel diagnostics and therapies by using tools provided by Computational Medicine. Similarly, Biology is advancing at a great pace with assistance from Computational Biology. In our own industry, Computational Finance is becoming the main engine of progress in a field that is increasingly algorithmic and data-driven.
One of the most important drivers for the emergence of a new engineering discipline is the rapid convergence of the Quantitative and the Computational domains. The role of Quant-developer has become recently one of the most looked-after specialties in the financial industry. One could see it as “two experts for the price of one” - a solid quant and a very capable software engineer in one person.
Because the quant space has become so much more competitive, the recruiting process has also changed accordingly. The interviewing process is a lot more challenging, requiring better preparation and a lot of stamina to be able to cope with the load and the complexity of the tests required.
2.2 Rigor and Practicality
Combining scientific rigor with engineering practicality has been the cornerstone of technological progress. The process of scientific research starts with formulating the problem and asking all necessary questions to help generating a hypothesis or a model. This process involves a careful analysis of the phenomenon under study and it requires a rigorous dose of skepticism of what is observed and measured (data).
Based on the data collected via observations, a hypothesis is then constructed and tested via experimentation. Since generality is always desirable, the reproducibility of results is tested under varied conditions.
The art of asking the right questions is a critical phase of the creativity process. In general three classes of questions are posed: the WHAT (objective), the WHY (causality or correlation) and the HOW (implementation). Business generally deals with planning and prioritization (the WHAT), Science offers the proper set of methods and techniques for solving the problem at hand from a theoretical perspective (the WHY), while Engineering provides the tools and processes to implement a practical solution (the HOW). This division of roles is going to get a lot fuzzier in the very near future, as the engineer of the 21st century will have to perform many of these tasks in rapid succession.
In the newly emerging paradigm of technological development, theory, experimentation, and simulation are used in a consolidated fashion to solve the kind of difficult practical problems that could not have found solutions until very recently [1]. An unintended consequence of this new paradigm is represented by the hype surrounding the so-called Artificial Intelligence revolution.
The term AI has become the mantra of our time as this label is used more and more frequently as an intellectual wildcard by academicians and practitioners alike. History showed as quite common the fact that a certain level of misunderstanding surrounds novel technology concepts when they are leaving the research labs and are crossing into the public domain.
The current AI-hype comes down to the idea that we are living in an era where the emergence of in-silico intelligence could compete with human intelligence. This is very misleading indeed and actually quite untrue. The success of this new engineering discipline will depend very much on whether or not we will be able to clarify the AI terminology and to adjust the expectations accordingly. Whether or not Science will be able any time soon to understand and properly model the concept of Intelligence, enrolling both computers and humans into the fight to enhance human life is a major challenge ahead!
Solving the challenge of understanding General Intelligence will be quintessential to the development of AI, and this new branch of engineering may be the catalyst for it. Like many other classic branches of engineering that emerged in the past (i.e. Civil, Mechanical, Electrical or Chemical), this new engineering discipline is going to be built on already mature concepts such as information, data, algorithm, computing, evolution, and optimization. Some may already have a name for this new discipline - Data Science.
However, in my opinion, this view is an oversimplified one, since there are a lot more components associated with this emerging discipline than just data.
No matter the label used, this new field will be focused on leveraging large amounts of data, intensive computations, and scientific knowledge to ultimately enhance human life. Therefore its development will require perspectives from a variety of disciplines: from quantitative sciences, like Mathematics and Statistics, to life sciences like Biology and Psychology, and from Computational Sciences to Engineering and Business.
2.3 Opportunities
The confluence of considerable scientific and technological progress created the conditions for the advent of what one could call Computational thinking. This concept originated more than a decade ago when Jeannette Wing [2] addressed the representation of Information (structured from Data) in an algorithmic way, such that on could objectify Knowledge for different problem domains. More recently Computational Intelligence emerged as a multidisciplinary framework that is focusing on problems that only humans can solve, problems requiring Intelligence.
The methods used by Computational Intelligence is close to human's way of reasoning, which is using inexact and incomplete information with the declared scope of generating controlled actions in an adaptive way.
There is a healthy competition between the science of Computational Intelligence, which is attempting to understand the principles of reasoning, and the engineering of Computational Intelligence, which focuses on coding the programs required to solve particular problems.
This duality is one of the main drivers of this discipline. The field of Computational Intelligence is aiming at the creation and testing of complex theories, specifically looking at how difficult practical problems could be represented and solved by a computer. These theories should have empirical support in real-world implementations, whose quality should be judged by traditional Computer Science principles. The main purpose of Computational Intelligence is to bridge the duality of specifying theories and building implementations [3].
3. Tools versus Problems
Computational thinking is perceived as an approach to solving problems rather than just another set of tools. Its purpose is to understand how intelligent behavior is possible, and the ultimate goal is to design, implement, and experiment with computational systems that perform tasks commonly viewed as intelligent.
I strongly believe that the development of problem-solving skills is far more important for the career advancement of a quantitative practitioner than the accretion and mastering of an ever-increasing set of new tools that are flooding both the technical literature and the higher education curricula. While the majority of these tools are becoming obsolete soon after their release into the public domain, acquiring an adequate level of problem-solving expertise will endow the learner with long-lasting know-how that will transcend ephemeral paradigms and cultural trends.
If the use of an exhaustive toolset is providing the solution architect with horizontal scalability, mastering the expertise of what tools to be used for any given problem will grant the user the vertical scalability that is absolutely necessary for implementing intelligent solutions. The relevance of this new breed of engineering for the financial industry resides on its practicality and in the primacy of developing problem-solving skills over the mastering of ephemeral tools.
4. A New Engineering Discipline
The success of the next industrial revolution will require a workforce that is engaged in a life-long cycle of learning. For this process to become efficient, it is necessary to create and adopt novel quantitative models that will continuously evaluate the efficiency of the learning process and develop the algorithms to optimize it.
Technology companies in general, and trading and asset management firms in particular need to gain the ability to have a quantifiable measure of the in-house resident knowledge, as well as a framework of well-defined metrics to be used in the recruiting process to seamlessly match business needs to talent.
Financial firms are looking for candidates that are mastering an ever-increasing set of tools, from Financial Mathematics to Computer Science, from Big Data Analytics to
Machine Learning and from
Blockchain to Quantum Computing technology. But no matter how extensive is the toolset, what they are especially looking for, is the ability of candidates to solve practical problems.
This brave new world will require a new breed of engineering workforce: one combining classical quantitative skills with deep knowledge of Computer Science and hands-on knowledge of modern HPC technologies. Since the central objective is
Innovation, fluency in the triad
Science-Engineering-Technology is a hard-core prerequisite that requires engaging in a life-long cycle of learning.
This new engineering discipline will be very different from all its predecessors because it will be
Data-driven,
Computationally-intensive,
Biology-inspired, and
Human-centered at the same time.
5. Conclusions
The recent technological progress has generated a set of very high expectations about the possibilities of using machine intelligence in every walk of life. There is a futuristic school of thought predicting that humanity will soon reach a singularity point in its development [4] when humans assisted by technology will transcend biological laws. But even if this improbable, far-fetched view that singularity point is ever going to be reached, what kind of workforce will be deeded to implement the new scientific theory into real-world products and services? Who is going to build the robots that will bring us to the singularity point?
Realistically, what kind of engineers is going to be able to carry on this work to create intelligent machines that could replicate themselves? Or even more realistically, how are business decision-makers going to select the right tools for their given problems? Such a bold disruption at a planetary scale will require novel approaches to educate the future workforce and to train the existing personnel.
Until such disruptive scientific and technological breakthroughs will occur, the best course of action is to continue to make steady progress on automating some of the most complex tasks at hand and to devise new ways of learning from data by using the computational toolset already available. At the same time, our economy will need to redirect its focus on the education process of the future workforce, and this will require mastering ever more complex tools and frameworks, and especially the development of solid and scalable problem-solving skills.
I will conclude outlining my own view on the mission of this new engineering discipline: nurture an innovative Engineering Mind that is driven by a powerful Mathematical Engine, supported by a vigorous Research Impetus and guided by strong Technology expertise. All of these components will need to coexist and to be nourished with up-to-date knowledge in order to properly develop the new breed of an engineer of the 21st century.
This new profession will represent a significant evolution in respect to the quant of the 20th century, as it will address the needs of the modern economy by making itself available to a variety of industries, way beyond the realm of the Finance. Fields like Medicine, Healthcare, Education and the Internet-of-Things are going to be big consumers of this novel specialty. Since the modern society is so immersed and dependent on data and the methods to extract actionable information from it, this new breed of engineers will position themselves at the core of the system that drives the most important business decisions.
Let’s be realistic and accept the fact that humanity’s most valuable asset is human Intelligence! Today our most important objective as quant educators is to kindle the interest in innovation and to nurture the problem-solving skills of our students.
The expectation is that this new engineering discipline will fulfill a very important societal role in facilitating the success of the next industrial revolution!
6. References
[1] Jim Grey on eScience: A Transformed Scientific Method, In Tony Hey, Stewart Tansley, and Kristin Tolle (Eds.) The Fourth Paradigm: Data-Intensive Scientific Discovery, Microsoft Research, 2009. Content available at: http://itre.cis.upenn.edu/myl/JimGrayOnE-Science.pdf
[2] J. Wing: Computational Thinking published in Comm. ACM, vol. 49, no. 3, 2006
[3] C. Doloc: Applications of Computational Intelligence in Data-Driven Trading, Wiley, 2019
[4] R. Kurzweil: The Singularity is Near: When Humans Transcend Biology, Viking Press, 2005
→ If you enjoyed reading this blog article, check Cris Doloc's previous article entitled: "Defining Learning in the context of Artificial Intelligence".